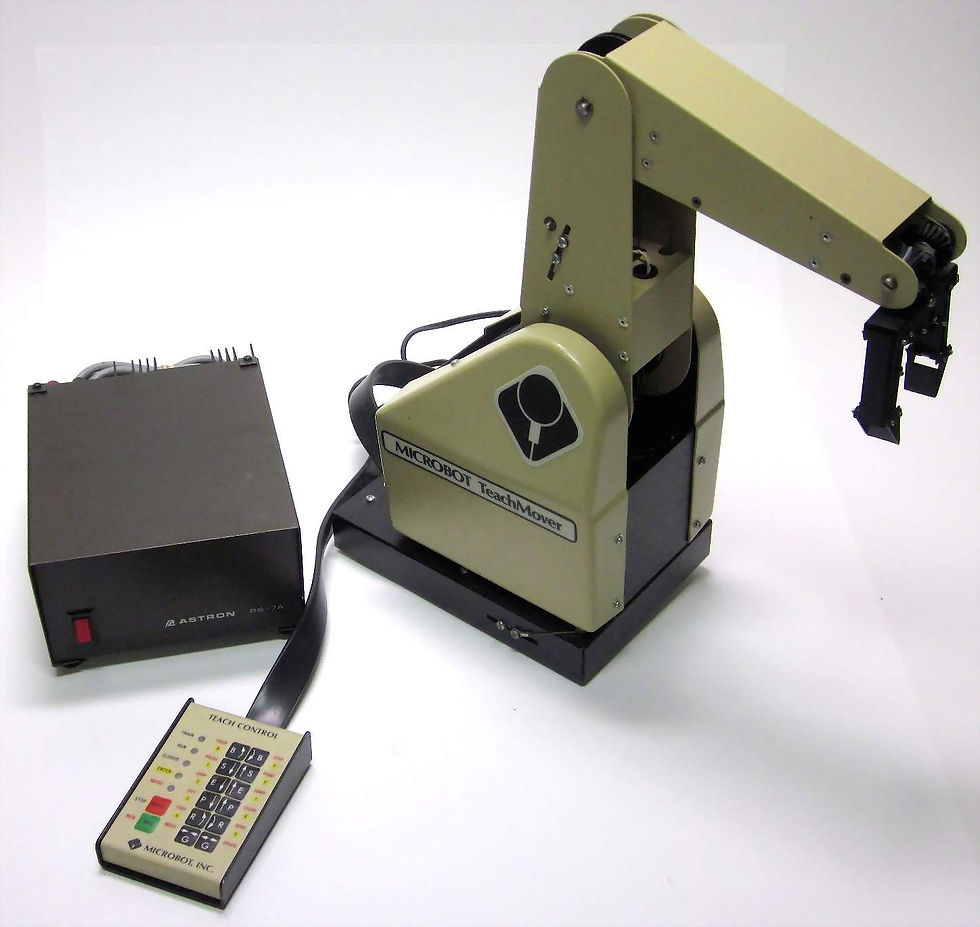
MCRobot [Latest] MCRobot Torrent Download is intended to be a toy application that serves as a platform to demonstrate the MCMC methods, using examples taken from Bayesian statistics. More... The JoVE video player is compatible with HTML5 and Adobe Flash. Older browsers that do not support HTML5 and the H.264 video codec will still use a Flash-based video player. We recommend downloading the newest version of Flash here, but we support all versions 10 and above. Markov chain Monte Carlo (MCMC) is a method of sampling which combines the strengths of Markov chain and Monte Carlo sampling by modelling with a Markov chain, and thereby avoiding the exponentially costly step of evaluating the partition function in a direct Monte Carlo estimator. MCMC is widely used and accepted in all fields of statistical theory, e.g., Bayesian statistics, econometrics, statistics, physics, chemistry, genetics, and linguistics. It is the essential tool in almost all of these fields. Background of MCMC The fundamental assumption of MCMC is that the current state of the system is the result of the past and of the present only, provided the observations are infrequent enough (i.e., Markovian). This leads to a kind of discrete stationary distribution of the states. In its basic version, MCMC generates samples according to the current state (instead of the Markov chain used for simulating the transient behavior) and provides a connection to sampling theory. Since MCMC is effectively sampling the distribution, the quality of the samples obtained by the MCMC method depends on two factors: (i) The distribution to be sampled and (ii) the sampler algorithm (meaning that of the Markov chain). The Markov chain that is most commonly used for MCMC is the Metropolis algorithm. It consists of a series of states that are connected to each other via the Markov chain. At each step a new state is chosen at random and the acceptance probability is calculated according to the ratio of the target distribution at the new state and the current state. The acceptance probability is calculated by evaluating the ratio of the target probability at the new state and the current state. If this ratio is larger than a defined threshold (the Monte Carlo accepted probability), the new state is accepted. Otherwise, the new state is accepted with a random (and very small) probability. As a side benefit, Markov chains are well known to be equivalent to Gibbs sampling, wherein a new state is chosen at random according to the current MCRobot Crack Full Version * Uses a Monte Carlo based statistical sampling algorithms for simple Bayesian modelling. * Runs MCMC (Markov Chain Monte Carlo) with the Metropolis-Hastings (M-H) algorithm * Totally FREE to use. No registration or sign up is required! No guarantee of accuracy. * Based on the principle of "simulated random walk" with normal distribution (see Equation 1) (Boos and Kalisch, 2012) * Suppose that the time t and your position x(t) are represented by the following Bayesian statistical models: x(t+1)=x(t)+V(t), x(0)=0 (see eq.2) x(t)=\int_{ -\infty}^{x(t)}g(x(t),p)dx(t)+\eta (1-g(x(t),p)) \eta\sim N(0,1) (see eq.3) Applying the Bayes rule on the last expression, you get: p(x(t)|x(0))=\frac{g(x(t),p)p(p)}{\int_{ -\infty}^{x(t)}g(x(t),p)p(p)dx(t)}=\frac{g(x(t),p)p(p)}{\int_{ -\infty}^{\infty}g(x(t),p)p(p)dx(t)}. x(t+1)=\int_{ -\infty}^{x(t)}g(x(t),p)dx(t)+\eta (1-g(x(t),p)) \eta\sim N(x(t),\frac{1}{2}), \eta\sim N(0,1) (see eq.4) Applying the Bayes rule you get: p(x(t)|x(t+1))=\frac{g(x(t),p)p(p)}{\int_{ -\infty}^{\infty}g(x(t),p)p(p)dx(t)}\times\frac{g(x(t+1),p)p(p)}{\int_{ -\infty}^{x(t)}g(x(t),p)p(p 09e8f5149f MCRobot Crack+ X64 MCRobot is a simulation program using the Monte Carlo (MC) method to perform random walks in space and time, for the purposes of simulating models, fields or data. It is for the most part, in the spirit of Metropolis-Hastings, but with a twist - the twist being that the method that MCRobot uses for random walks is the same as the one that you would use if you wanted to compute the Metropolis-Hastings ratio by hand! The twist: Since MCRobot is based on the pure MC method, which has an intrinsic ability to "auto-genuinely" find the 'hottest area' of the state-space, MCRobot is able to achieve high acceptance ratios with little effort. To achieve this trick, you simply give MCRobot access to your data, and let it go at it. This is the same as if you were just using the data to "train" a parameter. However, since MCRobot can take any data that you want, you can try different ones, or give MCRobot access to tricky data where the likelihood of acceptance is extremely low. To be clear, the features of MCRobot do not depend on whether you are doing Bayesian analysis or not. It is simply a simulation program that uses the Monte Carlo methods as its underlying foundation. This is a very powerful feature for those doing Bayesian analysis, since you can use MCRobot to test an overall parameter of the MCMC, namely, the MCMC burn-in time. Moreover, MCRobot can help you to predict when the MCMC will start to 'breed' false regions in the state-space, so you can decide whether you need to 'jump' out of the region or not. MCRobot Requirements: MCRobot is written in C and is compiled using gcc. It is written entirely in the C programming language and makes heavy use of the C library functions. It also makes use of the C++ library functions to make handling of objects simpler. Any version of C or C++ compilers can be used to compile MCRobot. MCRobot has no external dependencies, and can be compiled using any C/C++ compiler that includes a C compiler. MCRobot includes a long list of other projects that are freely available for use on all platforms. You can download it here: What's New in the? ---------------------------------------------------- MCRobot is a simulation tool for Bayesian analyses based on the Markov Chain Monte Carlo (MCMC) technique. Its purpose is to provide the researcher with a quick understanding of the theory underlying this statistical technique, along with a first-hand experience in its application. However, the tool is somewhat less useful for learning the theory for the first time, and it is designed to solve this specific problem. MCRobot is extensively discussed in the book by Theobald and Beaumont (1997). MCRobot Features: ------------------ The MCRobot feature set is included with this distribution (based on a Linux system), with the following modules: *** PDF of the MCRobot website. *** This is the first version of the MCRobot website. There are 28 pages, and there are 2 mailing lists available. *** Email of a list of news items from the mailing list. *** The news items on the mailing list summarize the latest development in statistical software and they are also available from the MCRobot website. *** Email of a list of news items from the MCRobot mailing list. *** The news items on the mailing list summarize the latest development in statistical software and they are also available from the MCRobot website. *** PDF of the MCRobot book. *** The book by Theobald and Beaumont (1997) is included with the distribution. This book is the first in a series of three books introducing the Markov Chain Monte Carlo methods. It is written to be accessible to a wide audience. *** PDF of the MCRobot manual. *** This manual was written by the developers of MCRobot. It explains all the concepts that MCRobot is based on. It is written in an easy-to-understand and understandable way, therefore it should be a valuable resource for anyone who wants to use MCRobot to learn the theory. The printed version of the manual is also included with the distribution. *** HTML of the MCRobot website. *** The website has 27 pages. The pages include basic pages for getting started, customization, and the capability table. They also include pages for the mailing lists, news items, and the manual. *** HTML of the MCRobot mailing list. *** System Requirements For MCRobot: CPU: Intel Core i3 or higher RAM: 4 GB or higher HDD: 60 GB or higher GPU: AMD HD graphics card or higher Windows: Windows 8.1 or higher Mac OS X: macOS 10.10 or higher Install instructions: Launch Steam Select "Play" from the dropdown menu Select "Activate a free game" Select the activation code provided Select "Install" from the dropdown menu
Related links:
https://secretcs2020.com/wp-content/uploads/2022/06/darlrai-1.pdf
https://arlingtonliquorpackagestore.com/maya-crack-torrent-activation-code-download-3264bit/
https://techfaqs.org/app/photo-stitching-software-pro-crack-product-key-download-2022-new/
http://srilankapropertyhomeandland.com/wp-content/uploads/2022/06/WinNetworkInfo.pdf
コメント